Y Hat Minus Y Bar
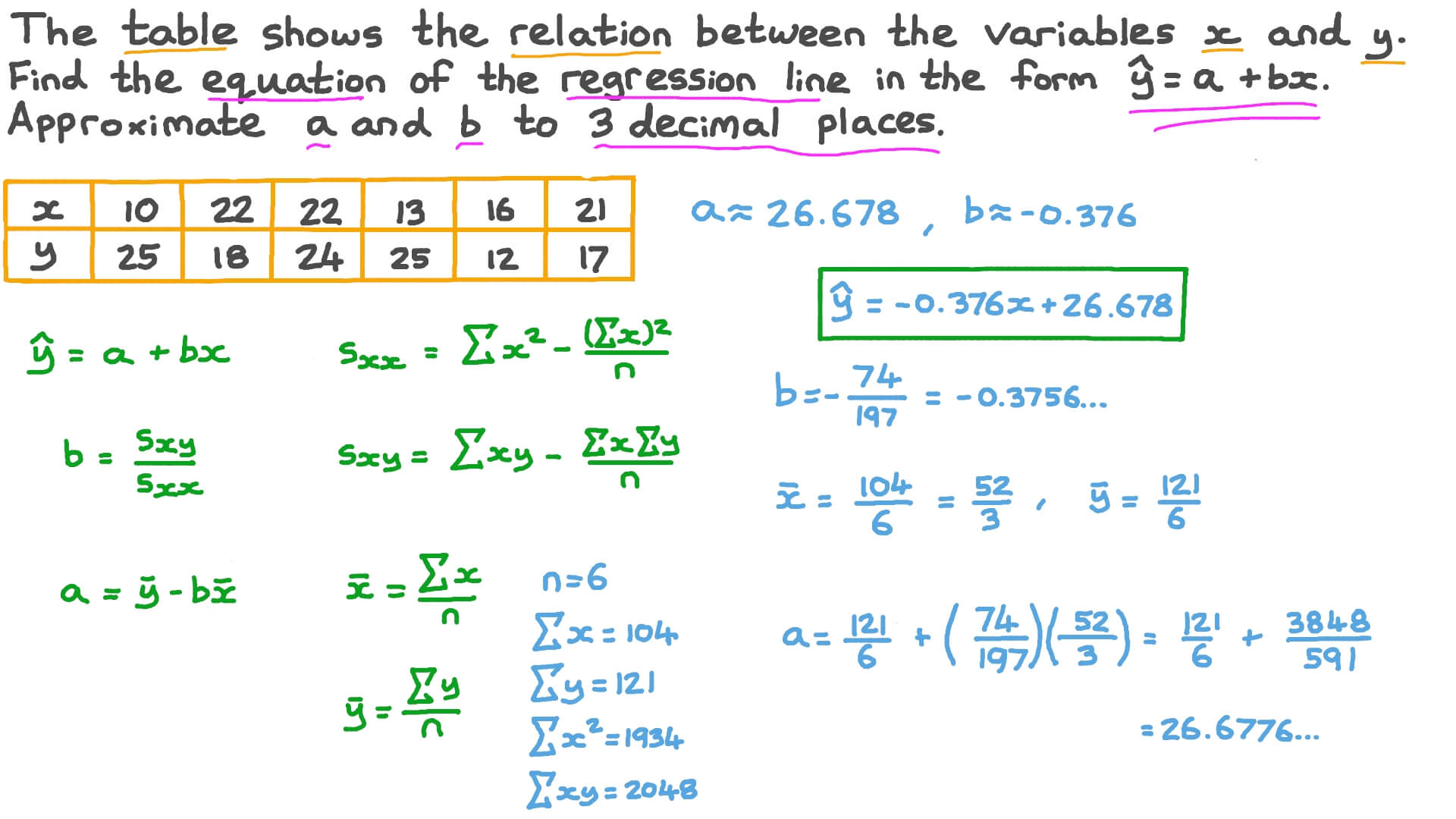
Thus I can divide both sides by n minus 1 the numerator both both the numerator and the denominator by n minus 1.
Y hat minus y bar. The residual symbolized by e-sub-I equals the data point y symbolized by y-sub-I minus the predicted value from the least-squares regression line symbolized y-hat. Y bar is how you read the symbol y. That summation yi minus y bar times xi minus x bar divided by summation xi minus x bar squared.
Now consider Covbarepsilon hatbeta_1 Ebarepsilon hatbeta_1 Covsum c_i epsilon_i barepsilon sum_i1n sum_j1n c_itimes dfrac1n Covepsilon_i epsilon_j textby independence we get sum_i1n dfracc_in sigma2 dfracsigma2nsum_i c_i 0 This is because c_i dfracX_i - barXsum_j1n X_j - barX2 sum_i1n c_i. Sum of square means equals the sum of the centriod symbolized by y-bar minus the predicted value of each x data point symbolized by y-hat sub I. Defined here in Chapter 3.
For bar use 0305 for hat. When working with a prediction model like a linear regression there are a few Ys you need to concern yourself with. Z standard score or z-score.
If so remember a very important property of this estimator is that the MMSE estimator of Y is that the error hat Y - Y is orthogonal to any function of Y. For this data Y Selling Price. Also let Varε Σ be an arbitrary symmetric positive semi-definite matrix.
I understand that this plot is conventionally used to test for constant variance. Y-hat ŷ is the symbol that represents the predicted equation for a line of best fit in linear regression. The residual plot std res vs the regression fits looks good.
Y-hat lowercase y with a caret circumflex b_0 b_1 x. Y hat minus y bar. The ys observed outcome variable the y-hats predicted outcome variables based on the equation and the residuals y minus y-hat.